Have you already an account ?
You are now registered for the ICFSR 2019 Congress which will take place in Miami Beach (USA) 20-22 February 2019
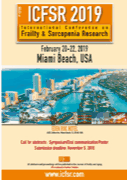
This entitles you to a free 1-year subscription for the online version of JFA magazine.
Create a new account
Customer account
You need to get a customer account in order to buy single articles from the The Journal of Frailty & Aging.
Individual Article (Electronic only) 40.00 EUR
Customer account
One year subscription
Register online now and immediately gain access to all articles from the The Journal of Frailty & Aging.
- Institution 925 EUR (Pharmaceutical industry, library, etc.)
- Individual 385 EUR
One year subscription